What is a specific data point, and why is it critical to analysis? A thorough understanding of individual data points is fundamental to accurate interpretation and informed decision-making.
A specific data point represents a single, measurable observation within a dataset. This could be a customer's purchase amount, a machine's operating temperature, or a student's test score. Each point contributes to the larger picture, but understanding their individual characteristics is crucial. For instance, in a dataset of sales figures, each individual sale amount (representing a unique transaction) is a data point. Analyzing these individual points can reveal trends, outliers, and correlations that might otherwise remain hidden within the aggregate data.
The significance of individual data points lies in their contribution to comprehensive analysis. Identifying patterns, trends, and outliers within the dataset depends on scrutinizing these individual observations. Further, understanding the context surrounding each point (like time of purchase or product type) allows for a deeper and more nuanced interpretation. This meticulous examination of individual data points is essential for informed decision-making in various fields, including business, science, and healthcare.
Specific Data Points
Understanding specific data points is fundamental to effective analysis. Accurate interpretation relies on careful consideration of individual observations within a dataset.
- Measurement
- Accuracy
- Context
- Variation
- Correlation
- Trend identification
- Interpretation
Each data point, a singular measurement, contributes to the larger picture. Accuracy ensures reliable analysis; context provides understanding. Variation reveals patterns; correlation identifies relationships. Trend identification allows for prediction; interpretation leads to informed decisions. For instance, a retailer analyzing sales figures would focus on the accuracy of each transaction, the context of the purchase (time of year, promotions), and variations in product sales to identify correlations and trends. This rigorous examination of individual data points is crucial for insightful interpretation and effective decision-making across many fields.
1. Measurement
Accurate measurement is fundamental to understanding specific data points. Precise and reliable measurement directly influences the quality and validity of subsequent analysis. Inaccurate or inconsistent measurements can lead to misleading conclusions and flawed interpretations. Consequently, rigorous attention to measurement methodology is essential for deriving meaningful insights from data.
- Precision and Accuracy
Measurement procedures must prioritize precision and accuracy. Precise measurements yield consistent results under repeated trials. Accuracy ensures the measurement reflects the true value of the phenomenon being studied. For example, in a study examining student performance, accurate measurement of test scores is crucial. Inaccurate measurements can skew results and distort the understanding of student performance. The precision of measuring instruments and the consistency of measurement techniques directly impact the reliability of data points, allowing for meaningful comparisons and conclusions.
- Unit of Measurement
Choosing the appropriate unit of measurement is critical. A lack of standardization in units can lead to misinterpretations and inaccuracies in analysis. For example, if a manufacturing process monitors temperature in both Celsius and Fahrenheit, comparing data from different periods becomes complex without a consistent unit. Selecting the correct unit for measurement ensures data can be meaningfully compared and analyzed across diverse scenarios.
- Instrumentation and Calibration
The tools used for measurement, such as scales, thermometers, or software, need proper calibration and maintenance. Malfunctioning or miscalibrated instruments can introduce error into data, compromising the validity of data points. Maintaining consistent calibration across measurements is essential to ensure the reliability of the data. For example, a faulty scale can lead to inaccurate measurements of product weights during manufacturing, which can have significant cost implications.
- Contextual Factors
Measurement must take into account the specific circumstances surrounding the data collection process. Environmental conditions, procedural variations, and observer bias can all affect measurements. Understanding the factors impacting measurement assists in identifying and mitigating potential sources of error. For example, measuring plant growth in different environments necessitates careful consideration of factors like light exposure, soil composition, and watering patterns. This contextual awareness enhances the accuracy and validity of the collected data points.
These facets of measurement underscore the importance of meticulous attention to detail in collecting data. By focusing on precision, standardization, instrument calibration, and contextual awareness, data collection procedures yield reliable data points. This, in turn, enables more accurate analysis and valid conclusions.
2. Accuracy
Accuracy in data collection and analysis is paramount for the reliability of any derived conclusions. This is particularly critical when working with specific data points, as the validity of subsequent interpretations hinges on the precision and truthfulness of each individual observation. Inaccurate data points can lead to flawed conclusions and ultimately, poor decision-making.
- Measurement Precision
Precise measurement techniques are essential for accurate data collection. Errors in measurement tools, inconsistent application of procedures, or observer bias can all introduce inaccuracies. Consider a study measuring the weight of manufactured components. Slight variations in weighing scales, differing operator techniques, or environmental factors influencing the scale can impact the accuracy of each measurement, potentially leading to flawed overall results. The accuracy of specific measurements directly influences the reliability of the final assessment of production quality.
- Data Integrity
Ensuring data integrity is crucial. Data entry errors, corrupted files, or inconsistencies in data formats can introduce inaccuracies. In clinical trials, each patient's recorded data pointblood pressure, weight, medication adherencemust be meticulously accurate to provide reliable evidence. Inaccurate data compromises the study's validity and the conclusions drawn about the treatment's effectiveness.
- Contextual Awareness
Data should always be interpreted within its proper context. Environmental conditions, time factors, or external variables influencing the observed phenomenon must be considered. A researcher studying plant growth might account for sunlight, soil moisture, and temperature in each measurement. Ignoring context can result in inaccurate conclusions about growth factors and potential interventions.
- Calibration and Validation
Regular calibration and validation of measurement instruments are necessary to maintain accuracy. In environmental monitoring, air quality sensors need regular calibration to ensure accurate readings. Ignoring this crucial step can lead to inaccurate assessments of pollution levels and hinder effective mitigation strategies.
In summary, maintaining accuracy at each stage of data collection and analysis is fundamental to valid interpretation of specific data points. Whether in manufacturing, healthcare, or environmental studies, the quality of conclusions hinges on the meticulous attention to detail and precision in the handling of data, ensuring accurate measurements, data integrity, and awareness of contextual influences.
3. Context
Context profoundly shapes the meaning and significance of any data point. A seemingly insignificant piece of data, a single data point (SD point), gains substantial meaning when considered within its surrounding circumstances. Isolated, a data point might be meaningless; contextualized, it becomes a crucial element of understanding a phenomenon. Understanding the context of a data point is essential for avoiding misinterpretations and drawing valid conclusions. For example, a sudden increase in sales might signal a positive market trend, or it could be an anomaly stemming from a temporary promotion. Without understanding the contextpromotional period, competitor activity, or economic indicatorsthe data point's interpretation remains incomplete and potentially misleading.
The importance of context extends to various fields. In medicine, a patient's blood pressure reading (a data point) is meaningless without considering factors like recent medication, physical activity, stress levels, or underlying conditions. Similarly, in financial analysis, a stock's price movement (a data point) carries more weight when examined against broader market trends, industry news, or company performance reports. Contextual awareness is fundamental to the correct interpretation and application of data in these and many other domains. Failure to consider context can lead to incorrect diagnoses, ineffective strategies, and poor decision-making, as evidenced by the potentially disastrous consequences of ignoring contextual factors in economic forecasting or medical diagnosis.
In essence, understanding context is not merely an enhancement but a necessity for valid analysis of individual data points. By meticulously considering the surrounding circumstances, data points transform from isolated observations into meaningful components of a larger, richer understanding. The significance of each data point thus lies not only in its numerical value but also in its position within a broader framework of context. Failing to acknowledge this connection can lead to significant errors in judgment and a lack of actionable insights.
4. Variation
Variation, in the context of data analysis, refers to the spread or dispersion of data points around a central tendency. Understanding variation is inextricably linked to comprehending specific data points (SD points). A data point's meaning is significantly shaped by its relationship to the overall variation within a dataset. A data point significantly deviating from the typical variation might signal an outlier, an error, or a noteworthy phenomenon. For instance, a manufacturing process producing components with consistently varying dimensions requires analysis to determine the root causes and implement corrective actions. Identifying the range of acceptable variation in component size allows for quality control and prevents production issues. Furthermore, understanding the variation in a dataset is fundamental for statistical inferences. Variation in blood pressure readings among a sample of patients, for example, helps establish confidence intervals when drawing conclusions about a wider population.
The importance of variation as a component of individual data points cannot be overstated. Analyzing variation helps identify patterns, trends, and anomalies in data. This includes understanding the typical range of data values, identifying outliers, and understanding the inherent variability in the measured phenomenon. Real-world examples abound. Stock market volatility reflects the variability of share prices. Agricultural yields fluctuate based on weather patterns, soil quality, and other environmental factors, illustrating the inherent variation in natural processes. Acknowledging this variability in data is crucial for forming accurate judgments and avoiding erroneous conclusions.
Recognizing and analyzing variation within a dataset is essential for effective data interpretation. Failure to account for variation can lead to skewed interpretations and poor decision-making. Accurate predictions, robust models, and effective strategies require consideration of variation in the context of specific data points. This is particularly critical in applications such as quality control in manufacturing, predicting future outcomes in finance, and diagnosing anomalies in complex systems. Understanding the relationship between individual data points and the overall variation they exhibit is therefore fundamental for informed decision-making across diverse fields.
5. Correlation
Correlation analysis explores the relationship between two or more variables. In the context of individual data points (SD points), correlation reveals whether changes in one variable are associated with changes in another. This analysis is crucial for understanding patterns and dependencies within datasets, providing insights that extend beyond isolated observations.
- Identifying Relationships
Correlation analysis identifies whether a relationship exists between two variables, and if so, the nature of that relationship. A positive correlation indicates that as one variable increases, the other tends to increase as well. A negative correlation indicates that as one variable increases, the other tends to decrease. The strength of the correlation, measured by a correlation coefficient, quantifies the extent to which the variables are related. For instance, in sales data, a positive correlation might exist between advertising expenditure and sales volume, suggesting that increased advertising tends to be associated with higher sales.
- Predictive Power
Strong correlations often suggest predictive power. If a strong positive correlation exists between two variables, changes in one can be used to predict changes in the other. In stock market analysis, a strong correlation between the performance of a particular sector and a specific index can guide investment strategies. Understanding correlation enables predictions about future trends based on historical data.
- Identifying Outliers and Anomalies
Correlation analysis helps identify data points that deviate significantly from expected relationships. These outliers might signal errors, unusual circumstances, or important phenomena warranting further investigation. For example, in medical data, a significantly high blood pressure reading for a patient whose other vitals and lifestyle parameters do not exhibit correlating factors could be a crucial indicator needing further attention. Correlation helps highlight the unusual.
- Understanding Complex Systems
Correlation analysis is crucial in understanding complex systems where numerous factors interact. Identifying correlations can elucidate how different aspects of a system relate to one another. In environmental science, analyzing correlations between various pollution levels and meteorological factors can shed light on the complex interactions governing pollution distribution.
In summary, correlation analysis provides a powerful framework for understanding the relationships between individual data points. By identifying patterns and dependencies within data, correlation analysis facilitates predictions, highlights anomalies, and enhances our comprehension of complex systems. This, in turn, strengthens the value of each individual data point by providing context and meaning within the broader dataset.
6. Trend Identification
Trend identification is a critical component of data analysis, particularly when considering individual data points (SD points). Identifying trends within a dataset allows for the interpretation of patterns and the prediction of future outcomes. The relationship between trend identification and individual data points is essential in various fields, including business, finance, and science.
- Recognizing Patterns in SD Points
Trend identification begins with recognizing patterns in individual data points. A consistent upward or downward movement in a series of data points signals a potential trend. Analyzing SD points helps uncover these patterns, enabling a deeper understanding of the underlying factors influencing the dataset. For example, in sales data, a consistent increase in daily sales figures might suggest an upward trend, potentially due to promotional activities or an increasing consumer demand for the product.
- Identifying Outliers and Exceptions
While trends represent general patterns, individual SD points that deviate significantly from the trend may reveal crucial information. These outliers might indicate unusual events, errors in data collection, or shifts in underlying trends. Understanding both the trend and the outliers provides a more holistic view. In financial markets, a stock price that deviates sharply from a general downward trend could signify a potential turnaround or unforeseen news impacting the company.
- Predicting Future Outcomes
Identifying trends in SD points allows for the prediction of future outcomes. By analyzing the historical data, trends can be extrapolated to anticipate future values. Predicting future sales based on historical trends enables businesses to optimize inventory, allocate resources, and plan for future growth. Similarly, understanding trends in patient health data allows healthcare providers to anticipate potential issues and proactively address them.
- Improving Decision-Making
Trend identification, supported by the analysis of individual data points, directly enhances decision-making processes. Understanding current trends helps organizations or individuals make informed choices about resource allocation, strategic planning, and other critical decisions. In scientific research, identifying trends in experimental data guides further research, leading to a deeper understanding of underlying mechanisms.
In conclusion, trend identification fundamentally relies on understanding individual data points. Analyzing these points, recognizing patterns, identifying outliers, and extrapolating to predict future outcomes are all essential for effective trend analysis. This analysis, in turn, significantly contributes to informed decisions across various fields, showcasing the critical connection between individual SD points and broader trends.
7. Interpretation
Accurate interpretation of specific data points (SD points) is essential for deriving meaningful insights. The value of individual data points lies not just in their existence, but in the understanding they provide when interpreted within a broader context. Interpretation transforms raw data into actionable knowledge, enabling informed decisions and effective strategies. This section explores crucial facets of interpretation in relation to specific data points.
- Contextual Understanding
Interpreting SD points requires placing them within their proper context. Factors like time, location, and related variables influence the meaning of a data point. For example, a sudden increase in website traffic might be attributed to a marketing campaign or a news event. Without considering context, the interpretation of the increased traffic could be incomplete or misleading. A robust interpretation considers the interplay of various factors.
- Identifying Trends and Patterns
Interpretation involves identifying trends and patterns within a series of SD points. Successive data points exhibiting a consistent upward or downward trend suggest a pattern and potentially a predictable outcome. Detecting patterns allows for forecasting and informed decision-making. For instance, consistent increases in sales over several months might indicate a growing market trend, justifying investment in expansion strategies.
- Recognizing Outliers and Anomalies
Interpreting data involves recognizing outliers, or data points significantly deviating from the norm. These outliers may indicate errors, unusual circumstances, or potentially important phenomena. For example, an unusually high temperature reading in a manufacturing process might signal a malfunctioning machine or a need for maintenance. Correctly interpreting outliers often leads to prompt resolutions and prevents further problems.
- Statistical Analysis and Validation
Statistical analysis plays a vital role in validating interpretations of SD points. Methods like regression, correlation, and hypothesis testing provide frameworks for evaluating the significance and reliability of identified patterns. Such analysis strengthens the credibility and actionable nature of the interpretation. For example, statistical significance helps distinguish between random fluctuations and meaningful trends in research data.
In essence, interpreting SD points is not a simple process of observation but a complex interplay of contextual understanding, trend recognition, outlier identification, and statistical validation. These facets provide a robust framework for transforming raw data into valuable insights, enabling effective decision-making in various contexts. The quality of interpretation directly influences the value and reliability of conclusions derived from SD points.
Frequently Asked Questions about Specific Data Points
This section addresses common inquiries regarding specific data points (SD points). Clear and concise answers are provided to enhance understanding and facilitate informed decision-making.
Question 1: What constitutes a specific data point (SD point)?
An SD point represents a single, measurable observation within a dataset. It could be a customer's purchase amount, a machine's operating temperature, or a student's test score. Each SD point contributes to the larger picture, but understanding individual characteristics is crucial for comprehensive analysis.
Question 2: Why is accurate measurement of SD points critical?
Accurate measurement of SD points is foundational to reliable analysis. Inaccurate or inconsistent measurements lead to misleading conclusions and flawed interpretations. Rigorous attention to measurement methodology is crucial for deriving meaningful insights.
Question 3: How does context influence the interpretation of SD points?
Context significantly shapes the meaning of an SD point. Factors like time, location, and related variables provide crucial insights. Interpreting an SD point in isolation often yields incomplete or misleading conclusions. Understanding the surrounding circumstances is essential for accurate interpretation.
Question 4: What role do outliers play in the analysis of SD points?
Outliers, or data points significantly deviating from the norm, may indicate errors, unusual circumstances, or important phenomena. Their presence often signals a need for further investigation, enhancing comprehension of the data's complete picture.
Question 5: How does correlation analysis aid in understanding SD points?
Correlation analysis examines the relationships between two or more variables. By identifying correlations, analysts can understand patterns and dependencies within datasets, increasing the insights derived from SD points. Strong correlations often suggest predictive power, facilitating future projections.
Understanding specific data points (SD points) necessitates a multifaceted approach. Accuracy, context, trends, and correlations are key elements in extracting valuable insights from data. Accurate interpretation fosters effective strategies and decisions.
The following section delves deeper into the practical application of specific data points across various sectors.
Conclusion
This exploration of specific data points (SD points) underscores the critical role individual observations play in comprehensive analysis. Accurate measurement, careful consideration of context, recognition of variations, and the identification of correlations are fundamental to extracting meaningful insights from data. The significance of each SD point stems not only from its inherent value but also from its relationship within the larger dataset. A thorough understanding of trends, outliers, and potential relationships is essential for informed decision-making and effective strategies across various fields. Failure to account for these interconnected aspects can lead to flawed interpretations and ultimately, suboptimal outcomes.
The analysis of specific data points, therefore, necessitates a rigorous and multifaceted approach. By embracing precise measurement techniques, understanding contextual factors, and leveraging statistical tools, organizations and individuals can gain a deeper understanding of complex phenomena and make more informed decisions. The increasing availability and complexity of data necessitate a growing emphasis on the meticulous analysis of individual data points, ensuring a more nuanced and reliable understanding of the world around us.
You Might Also Like
Best Ullu Web Series List: Top Picks & New ReleasesDownload Ullu Web Series - Latest Episodes & Full Seasons
Best Movie Download Websites 2024
Best Vegamovies.do Alternatives & Streaming Sites
Free 300MB Movies - Download Now!
Article Recommendations
- Unveiling The Financial Journey Of Lewis Pullman What Is His Net Worth
- Decoding Glhf Meaning A Dive Into Gaming Culture
- Meet The New Paw Patrol Characters Discover Their Names And Roles

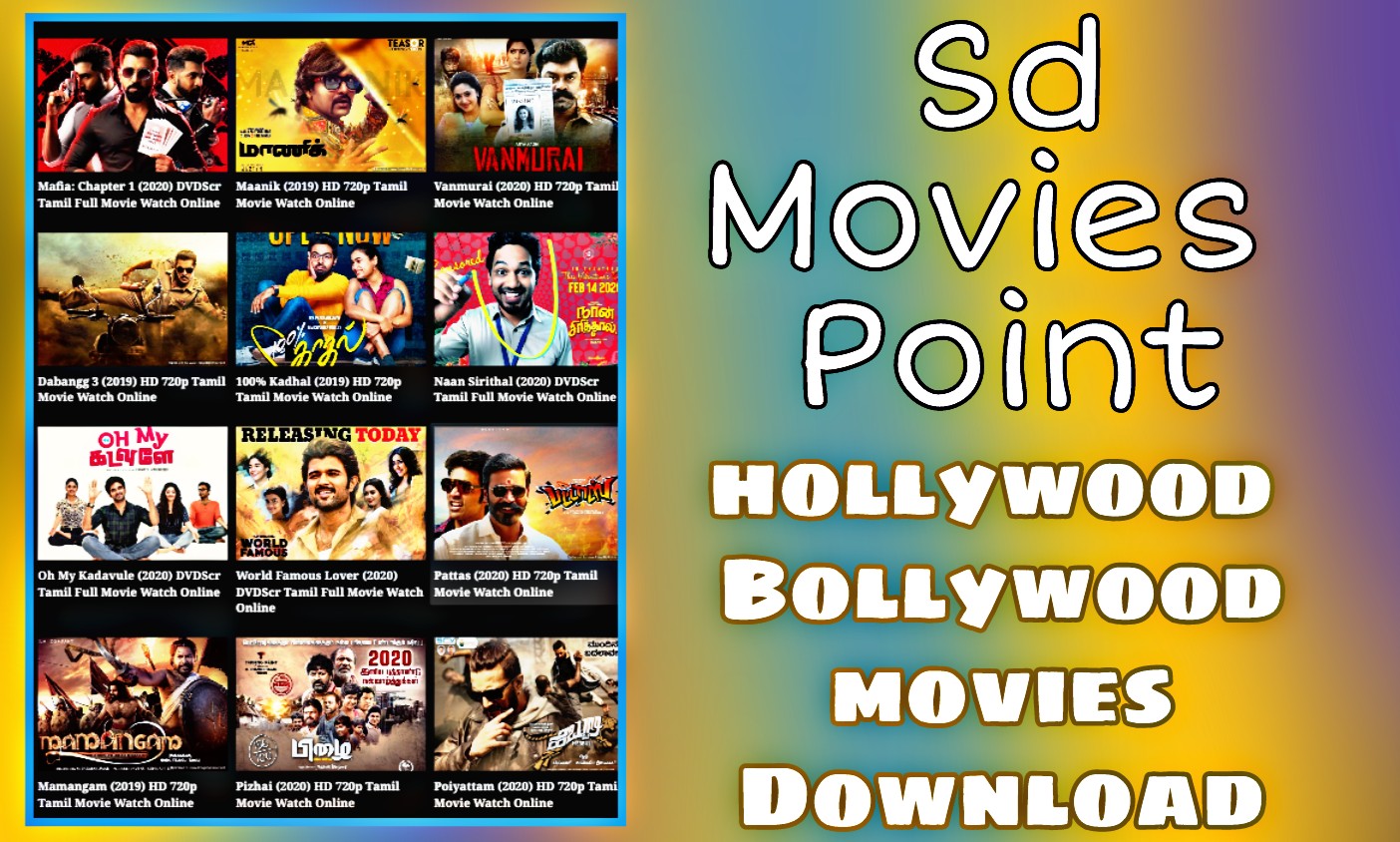
